Predicting Collective Evacuation from Wildfire (Chantal Nguyen, Kimberly Schlesinger)
Human decision-making is an important component of evacuation research, and understanding this complex factor could help make evacuations safer, swifter, and more effective, inform evacuation policies and multilayer traffic simulations, and provide much needed insight into possible alterations to public safety education and awareness campaigns.
To probe collective decision-making, we have conducted controlled behavioral experiments in which participants decide whether and when to evacuate from a virtual impending disaster in a series of different scenarios. We also examine mesoscale dynamics of collective decision-making by assigning participants to groups which act according to a specified protocol (e.g. majority-vote, or complete consensus) and where social pressure plays a role in the decision dynamics.
We develop a data-driven model that predicts collective population-level evacuation by defining a rule for individual decisions as a parsimonious function of disaster information (probability of disaster strike, and available shelter capacity). We use this model to elucidate the decision-making strategy shifts that occur when individuals act as part of a group and the effect of heterogeneity in individual preferences on group behavior.
In a second modeling approach, we develop a logistic regression model to predict the evacuation decision-making of individuals and groups, taking disaster information, and its variation over time, as input to the model. By including a measure of individual heterogeneity, obtained by aggregating data from participants' Facebook profiles, we improve prediction and achieve accuracy of close to 85%.
Past projects
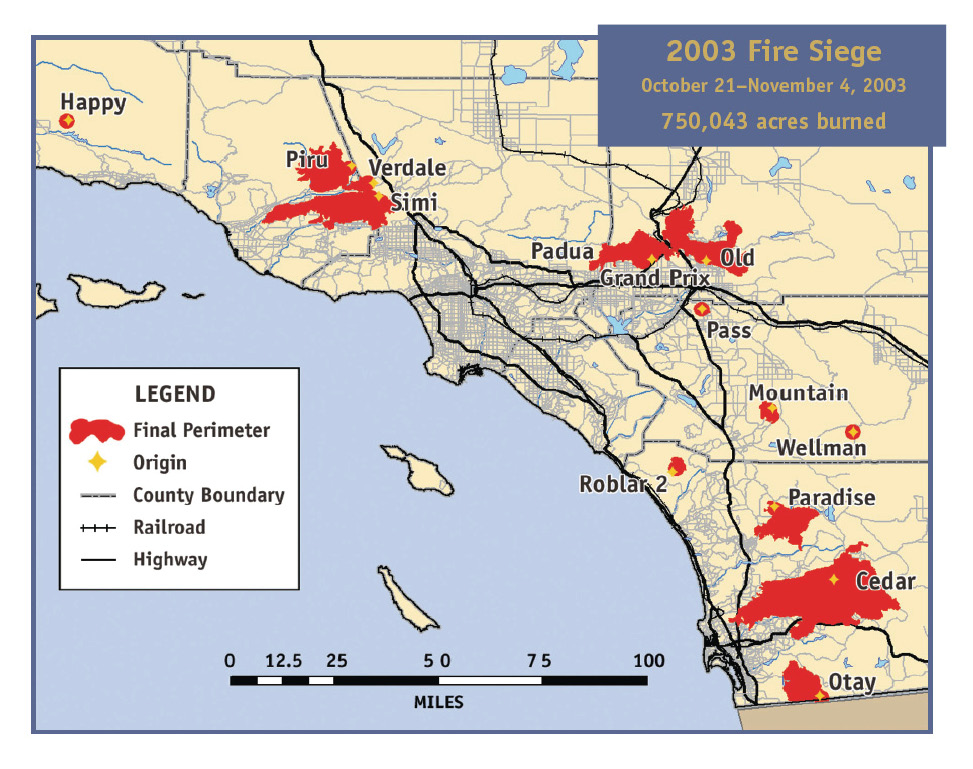
The work described below was performed in collaboration with David Alderson and Emily Craparo at the Naval Postgraduate School and was part of the much larger Multidisciplinary University Research Initiative (MURI) for Next Generation Network Science.
Wildfires and Disaster Response (Nada Petrovic)
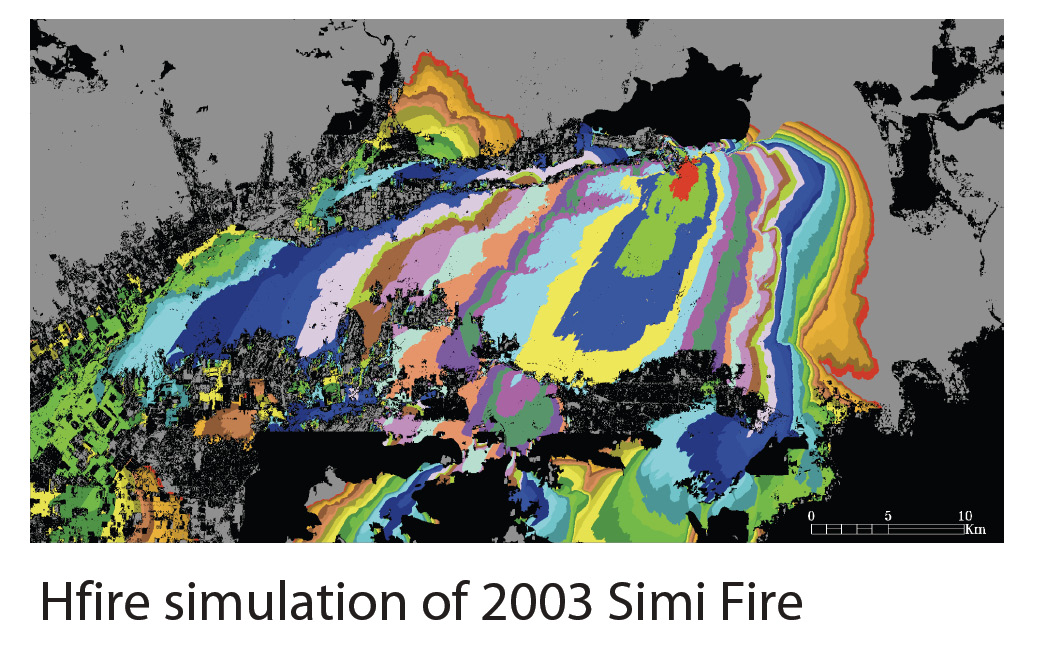
The economic, environmental and social impact of wildfires is an increasing problem in California and elsewhere. Suppression decisions present a complex resource allocation problem involving a combination of inputs. We examine tradeoffs which arise in wildfire response by combining a dynamic model of fire spread, an economic cost model, and population data. Statistical distributions of fire frequency versus size are generated using HFire, an efficient Rothermel based model for fire spread. Tradeoffs between resource costs and assets at risk determine the go versus no go decision for an individual fire. We also evaluate the cost of optimal and non-optimal strategies during simultaneously burning fires. Finally we compare our results with data from the 14 simultaneous fires of October of 2003 and the 16 of October of 2007 to consider the decisions made in real situations.
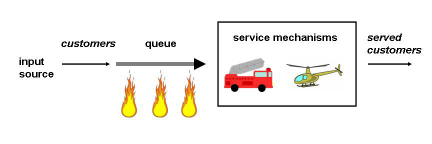
Wildfires and Disaster Response: Dynamics (Nada Petrovic)
Wildfire response demands dynamic decision tools because fires and suppression evolve simultaneously. Time delays can lead to larger fires and thus greater demand for resources. We capture this tension using a queuing model that treats fire progression as a birth and death process, with rates that incorporate intrinsic fire dynamics and suppression. Using this framework we explore tradeoffs in effectiveness and time of response, as well as implications for spatial prepositioning of resources.
Influence of Information Networks in Decision Dynamics (Danielle Bassett)
In this work, we model a population of individuals who receive information about an impending disaster from multiple sources, and must decide whether to stay (not evacuate) or go (evacuate). We are specifically interested in characterizing the interplay between global information dissemination (official news or mandatory evacuation orders) and social sharing, where information flows over a dynamic network of friends and neighbors.
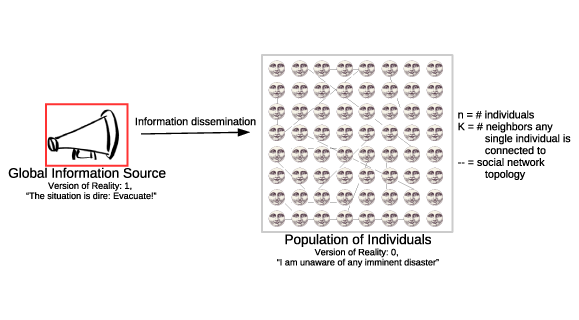
Publications
Modeling individual and group evacuation decisions during wildfires. C. Nguyen, K. J. Schlesinger, F. Han, I. Gur, J. M. Carlson. Fire Technology doi:10.1007/s10694-018-0770-7 (advance online publication), 2018.
Data-driven models for individual and group decision making. C. Nguyen, K. J. Schlesinger, and J. M. Carlson. In the Proceedings of the 2017 IEEE/ACM International Conference on Advances in Social Networks Analysis and Mining. doi:10.1145/3110025.3116204, 2017.
Collective decision dynamics in group evacuation. C. Nguyen, K. J. Schlesinger, and J. M. Carlson. In the Proceedings of the Summer Computer Simulation Conference 2017. doi:10.22360/SummerSim.2017.SCSC.002, 2017.
Measuring and modeling behavioral decision dynamics in collective evacuation. J. M. Carlson, D. L. Alderson, S. P. Stromberg, D. S. Bassett, E. M. Craparo, F. Guiterrez-Villareal, and T. Otani. PLOS ONE 9(2): e87380, 2014.
A decision making framework for wildfire suppression. N. Petrovic and J. M. Carlson, International Journal of Wildland Fire doi.org/10.1071/WF11140, 2012.
Collective decision dynamics in the presence of external drivers. D. S. Bassett, D. L. Alderson, and J. M. Carlson Phys Rev E 86:036105, 2012.
Dynamic resource allocation in disaster response: Tradeoffs in wildfire suppression. N. Petrovic, D. L. Alderson, and Jean M. Carlson. PLOS ONE 7(4): e33285, 2012.