Architecture of human white matter connectivity (Danielle Bassett)
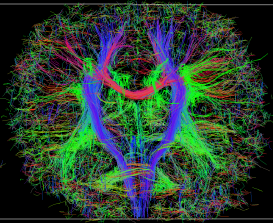
Recent advances in diffusion based magnetic resonance imaging (MRI) techniques and complimentary white matter tractography have made it possible to estimate the locations of anatomical highways spanning the cortex in the healthy and diseased human brain. Collectively, these deduced highways form a network architecture which, far from being random or haphazard, suggests that the cortex has precise internal structure. Studying this measured architecture may therefore provide potential insight into how macroscopic white matter structure both constrains and facilitates healthy cognitive function. In our work, we identify properties of network architecture which are conserved in the healthy human population and simultaneously characterize signatures of individual network variation. We are particularly interested in understanding how the topology of cortical connectivity was embedded into 3-dimensional physical space.
Learning and memory in neural networks (Ann Hermundstad)
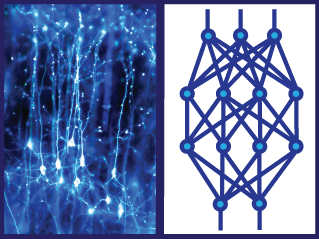
Neural networks are information processing structures that have been used for a variety of both computational and biological applications. The ability to train networks to learn and store complex patterns makes them a useful tool for studying the acquisition and retention of information. Of particular interest is the process of sequential learning, during which a network must integrate new information with previous knowledge. This requires the network to balance learning and memory, potentially invoking trade-offs between the two goals. Our work focuses on the role of network architecture in performing complex, sequential tasks, specifically comparing computationally and biologically motivated architectures. By understanding the influence of architecture on learning and memory, we hope to gain insight into the relationship between structure and function in the human brain. [Image Credit: Dr Jonathan Clarke. Wellcome Images, images.wellcome.ac.uk.]
EEG/fMRI (Kevin Brown)
![]()
The two best methods to obtain noninvasive, covert measurements of human neural activity are electroencephalography (EEG) and functional magnetic resonance imaging (fMRI); except in rare cases, they remain our only ways of mapping human brain activity. Unfortunately, the questions we can pose about human cognitive dynamics are limited by the technologies: EEG allows millisecond time resolution but only records from roughly 100 sensors on the surface of the scalp, and fMRI allows millimeter (~10,000 neurons) spatial resolution but only has time resolution of seconds, slow compared to timescales of interest in the brain. In order to obtain better 4D maps of human brain dynamics, and to use that information in generating and constrain
ing theoretical models of information processing in the brain, it is critical that we merge the best qualities of both EEG and fMRI to obtain simultaneously high spatial and temporal resolution. Concomitant with attempts to fuse EEG and fMRI data are fundamental questions about the limits of noninvasive imaging data. In what experimental situations do we benefit from vastly increased resolution? When do the data warrant a more complex model, for example physiological models of the fMRI signal or complicated geometries for brain, skull and scalp surfaces?